Domain Adaptation Covariate Shift
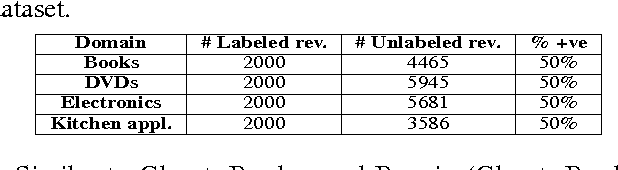
Cecotti li y g.
Domain adaptation covariate shift. A majority of domain adaptation algorithms rely on co variate shift while the rest mostly seek a common feature representation where covariate shift holds or have access to target labeled data. During my presentation on transfer learning i was asked the difference between covariate shift and domain adaptation. Abstract unsupervised domain adaptation aims to learn a model on a source domain with labeled data in or der to perform well on unlabeled data of a target domain. Application to brain computer interface proceedings of the 2015 international joint conference on neural networks ijcnn ieee 2015 pp.
All i know is that covariate shift takes place when data distribution changes over time and that this change in data distribution changes the original domain we were working in and that since our original domain has got changed or shifted we need to adapt our original. 1 domain adaptation under covariate shift we now shift focus again to a type of perturbation called covariate shift. Current approaches focus on learning.
Reliable uncertainty estimates are an important tool for helping autonomous agents or human decision makers understand and leverage predictive models. Prasadlearning with covariate shift detection and adaptation in non stationary environments. In this paper we introduce a domain adaptation algorithm that takes a different strategy based on a relaxed or probabilistic version of covariate. By covariate shift adaptation steffen schneider university of tübingen imprs is evgenia rusak university of tübingen imprs is luisa eck lmu munich.
Such unsupervised adaptation mechanisms are studied in the field of domain adaptation da which is concerned with adapting models trained on one domain the source here clean images to. Covariate shift is the change in the distribution of the covariates specifically that is the independent variables this is normally due to changes in state of latent variables which could be temporal even changes to the stationarity of a temporal process or spatial or less obvious. However existing approaches to estimating. Calibrated prediction with covariate shift via unsupervised domain adaptation.
Of all the manifestations of dataset shift the simplest to understand is covariate shift. We work in a classi cation or regression setting where we wish to predict yfrom x and make the assumption that p yjx and p yjx are the same the labeling function doesn t change between train and test.