Domain Adaptation Data Augmentation
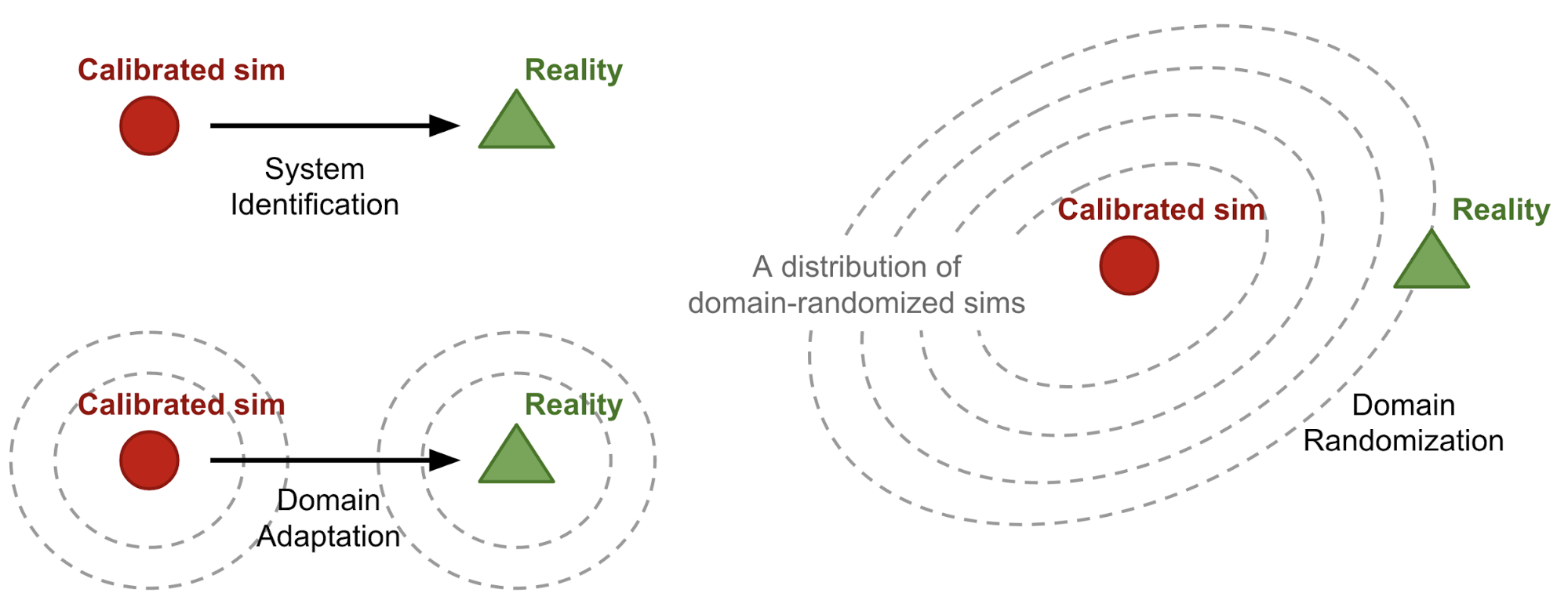
A number of authors have proposed domain adaptation methods for example see 9 39 36 26 40.
Domain adaptation data augmentation. Detection accuracy comparison ap detectors trained with trans formed images produced by gans trained with gt a dataset and. Any other ideas to do data augmentation for time series forecasting. Self ensembling with gan based data augmentation for domain adaptation in semantic segmentation jaehoon choi kaist taekyung kim kaist changick kim kaist whdns44 tkkim93 changick kaist ac kr abstract deep learning based semantic segmentation methods have an intrinsic limitation that training a model requires. Domain adaptation a case study in human activity recognition evaggelos spyrou 1 2 eirini mathe 2 3 georgios pikramenos 2 4 konstantinos kechagias 4 and phivos mylonas 3 1 department of computer science and telecommunications university of thessaly 35131 lamia greece 2 institute of informatics and telecommunications national center for.
Despite recent gan generative adversarial. Adversarial feature augmentation for unsupervised domain adaptation riccardo volpi1 pietro morerio1 silvio savarese2 vittorio murino1 3 friccardo volpi pietro morerio vittorio murinog iit it ssilvio stanford edu 1pattern analysis computer vision istituto italiano di tecnologia 2stanford vision and learning lab stanford university. Self ensembling with gan based data augmentation f or domain adaptation in. Kaist whdns44 tkkim93 changick.
However applying self ensembling to semantic segmentation is very difficult because heavily tuned manual data augmentation used in self ensembling is not useful to reduce the large domain gap in the semantic segmentation. Which doesn t cover forecasting however. Recent advances in big data systems and databases have made it possible to gather raw unlabeled data at unprecedented rates. Cross domain adaptation with gan based data augmentation 9 t able 4.
Adaptive data augmentation method where we add adversarially perturbed samples at the current model to the dataset section 3. Deep learning based image to image translation methods aim at learning the joint distribution of the two domains and finding transformations between them. However labeling such data constitutes a costly and timely process. I ve found the paper data augmentation for time series classification using convolutional neural networks by le guennec et al.
This is especially true for video data and in particular for human activity recognition har tasks. I m currently thinking about the same problem. Technologies article data augmentation vs. In this paper we introduce a self ensembling technique one of the successful methods for domain adaptation in classification.