Domain Adaptation Medical Imaging
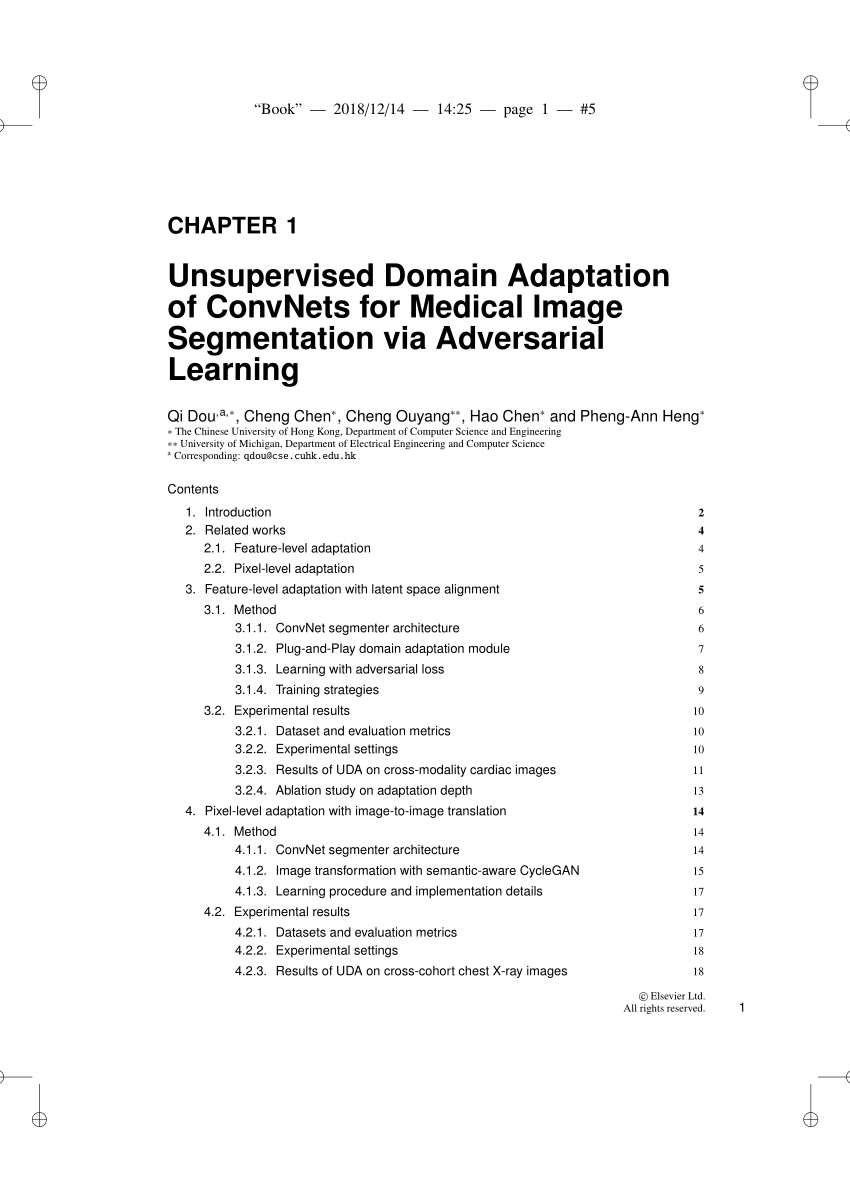
Barros b julien cohen adad a c show more.
Domain adaptation medical imaging. 4 share. Unsupervised domain adaptation for medical imaging segmentation with self ensembling article perone2019unsupervisedda title unsupervised domain adaptation for medical imaging segmentation with self ensembling author christian s. 11 14 2018 by christian s. Perone a pedro ballester b rodrigo c.
Recent deep learning methods for the medical imaging domain have reached state of the art results and even surpassed human judgment in several tasks. Unsupervised domain adaptation for medical imaging segmentation with self ensembling. Manual annotation is costly and time consuming if it has to be carried out. Those models however when trained to reduce the empirical risk on a single domain fail to generalize when applied on other domains a very common scenario on medical imaging due to the variability of images and anatomical structures even.
The work albadawy et al 2018 discusses the impact of deep learning models across different institutions showing a statistically significant performance decrease in cross institutional train and test protocols. 2019 a novel domain adaptation framework for medical image segmentation. Despite the advancement of machine learning in automatic segmentation performance often degrades when algorithms are applied on new data acquired from different scanners or sequences than the training data. Fast algorithms for biophysically constrained inverse problems in medical imaging.
Ballester and rodrigo c. Recent deep learning methods for the medical imaging domain have reached state of the art results and even surpassed human judgment in several tasks. Unsupervised domain adaptation for medical imaging segmentation with self ensembling author links open overlay panel christian s. Perone and pedro l.
As mentioned above one of the main challenges in medical imaging is the scarcity of training data. To address this issue we use a novel domain adaptation strategy and generate synthetic tumor bearing mr images to enrich the training dataset. The variations in multi center data in medical imaging studies have brought the necessity of domain adaptation. Barros and julien cohen adad journal neuroimage year.
Thesis the university of texas at austin 2017 google scholar. Recent advances in deep learning methods have come to define the state of the art for many medical imaging applications surpassing even human judgment in several tasks.