Domain Adaptation Papers With Code
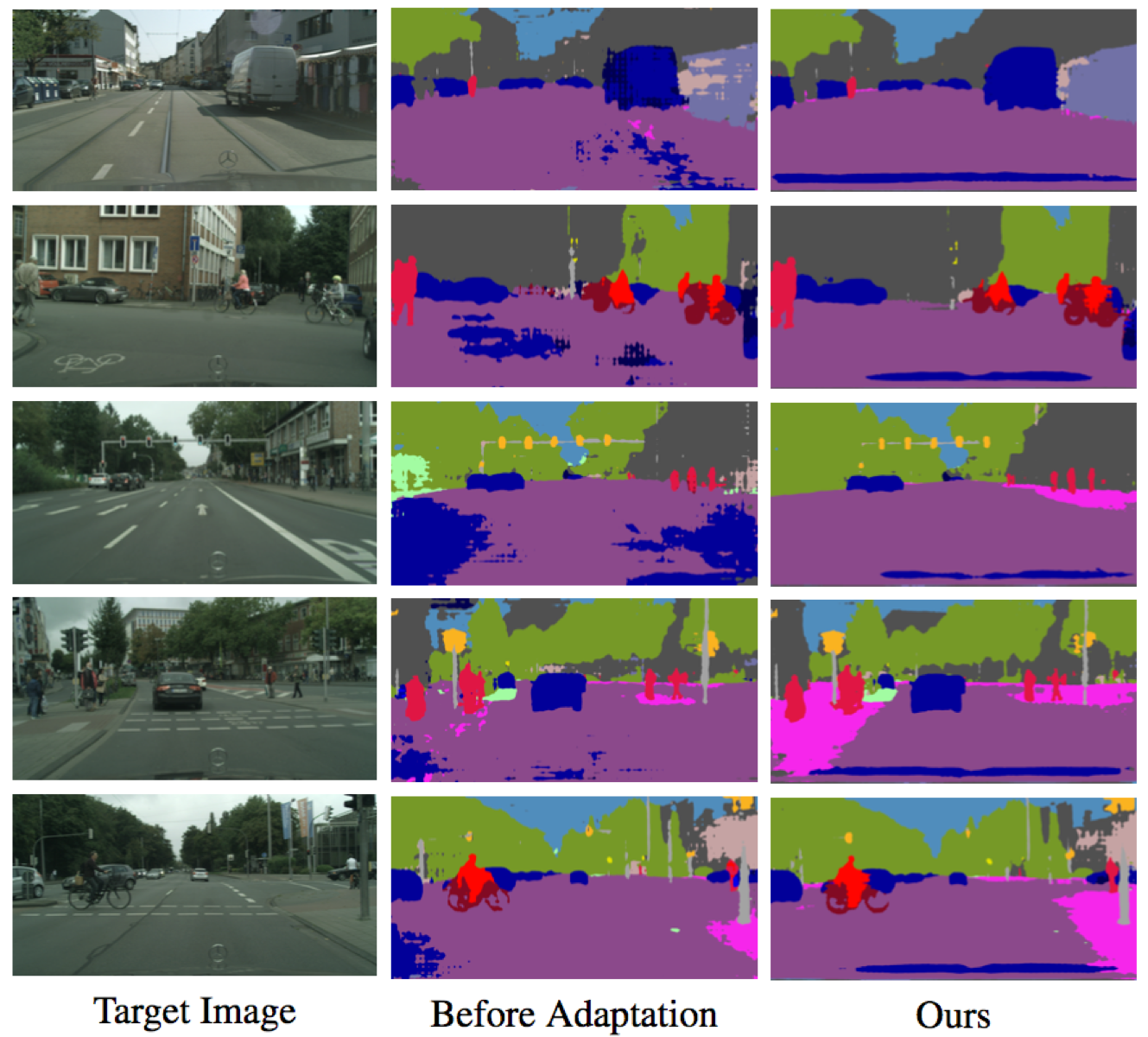
Unsupervised domain adaptation techniques have been successful for a wide range of problems where supervised labels are limited.
Domain adaptation papers with code. Contribute to barebell da development by creating an account on github. Domain adaptation papers and code. Software engineering best practices. Unsupervised domain adaptation papers and code.
Unsupervised pixel level domain adaptation with generative adversarial networks. After reading a few domain adaptation papers and their implementations i noticed a recurring pattern. Eccv 2020 bradyz task distillation we use these recognition datasets to link up a source and target domain to transfer models between them in a task distillation framework. The task is to classify an unlabeled target dataset by leveraging a labeled source dataset that comes from a slightly similar distribution.
We propose metric based adversarial discriminative domain adaptation m adda which performs two main steps. This paper surveys the advances and proposes a new taxonomy in transfer learning and domain adaptation for computer vision tasks in the past decade 127 lei zhang shanshan wang guang bin huang wangmeng zuo jian yang and david zhang manifold criterion guided transfer learning via intermediate domain generation arxiv 2019. Cvpr 2017 tensorflow models collecting well annotated image datasets to train modern machine learning algorithms is prohibitively expensive for many tasks. Domain adaptation with multiple sources.
Advances in neural information processing systems 21 nips 2008 authors. Domain adaptation through task distillation. This paper presents a theoretical analysis of the problem of adaptation with multiple sources. Domain adaptation addresses the problem of generalizing from a source distribution for which we have ample labeled training data to a target distribution for which we have little or no la bels 3 14 28.
Log bilinear language adaptation model we consider the domain adaptation problem from a source domain s to a target domain t. In the source domain we have l s labeled sentences xs i y s i l s i 1 and u.