Domain Adaptation Vs Fine Tuning
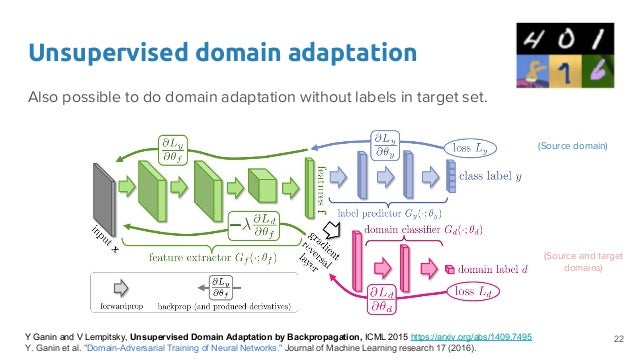
As you will notice this list is currently mostly focused on domain adaptation da and domain to domain translation.
Domain adaptation vs fine tuning. Day vs night synthetic data e g rasterization vs prof. Transfer learning or domain adaptation is related to the difference in the distribution of the train and test set. Transfer learning is commonly understood to be the problem of taking what you learned in problem a and applying it to problem b. Inspired by the need to create.
Rest assured these are all related and try to solve similar problems. In this paper we tackle the new cross domain few shot learning benchmark proposed by the cvpr 2020 challenge. Hence it is sometimes confusing to differentiate between transfer learning domain adaptation and multi task learning. As by using preinitialized weights instead of random ones you are in effect transfering knowledge from one domain to another.
So there s usually not. During breast cancer progression the epithelial to mesenchymal transition has been associated with metastasis and endocrine therapy resistance. A list of awesome papers and cool resources on transfer learning domain adaptation and domain to domain translation in general. It really depends on the context in which those terms are being used.
This is a more involved technique where we do not just replace the final layer. Domain adaptation is a field associated with machine learning and transfer learning this scenario arises when we aim at learning from a source data distribution a well performing model on a different but related target data distribution. To this end we build upon state of the art methods in domain adaptation and few shot learning to create a system that can be trained to perform both tasks. However the underlying mechanisms remain elusive.
Webcam model 1 vs webcam model 2. Fine tuning a pre trained network is a type of transfer learning. One could argue domain adaption is the correct term here but almost all the literature i ve seen calls it transfer learning via fine tuning or something similar. Fine tuning off the shelf pre trained models.
So it is something broader than fine tuning which means that we know a priori that the train and test come from different distribution and we are trying to tackle this problem with several techniques depending on the kind of difference instead of just trying to adjust some.