Domain Adaptation Vs Semi Supervised Learning
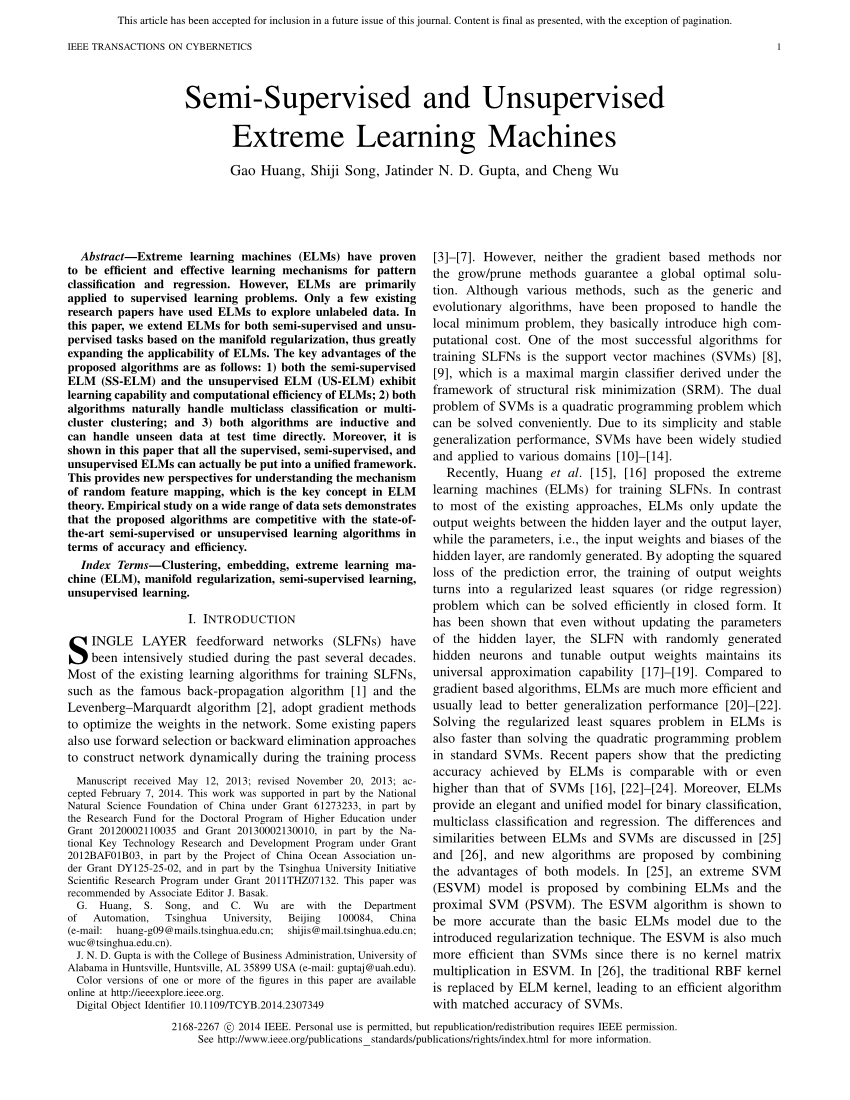
Semi supervised learning with localized domain adaptation and iterative matching qun liu matthew shreve and raja bala palo alto research center palo alto california abstract although data is abundant data labeling is expensive.
Domain adaptation vs semi supervised learning. We would then train a cla. Transferring knowledge from a source domain to a target domain by domain adaptation has been an interesting and challenging problem in many machine learning applications. We revisit this task and compare our approach to recent semi supervised learning or unsu pervised domain adaptation methods. Semi supervised learning methods combine a few labeled sam.
Semi supervised domain adaptation by covariance matching abstract. 0 share. For this classification task we need to first collect many reviews of the product and annotate them. Semi supervised domain adaptation with instance constraints jeff donahue1 2 judy hoffman1 2 erik rodner2 3 kate saenko4 trevor darrell1 2 1uc berkeley eecs 2uc berkeley icsi 3university of jena 4university of massachusetts lowell 1 jdonahue jhoffman trevor eecs berkeley edu 3erik rodner uni jena de 4saenko cs uml edu abstract.
Domain adaptation da is the topical problem of adapting models from labelled source datasets so that they perform well on target datasets where only unlabelled or partially labelled data is available. The main challenge in domain. Semi supervised domain adapta tion ssda is a very important task 8 40 1 however it has not been fully explored especially with regard to deep learning based methods. 04 09 2020 by da li et al.
Consider the problem of sentiment classification on reviews on a product such as a brand of camera. To address the above issues we propose a novel semi supervised representation learning framework via dual autoencoders ssrlda for domain adaptation.