Domain Adaptation Reinforcement Learning
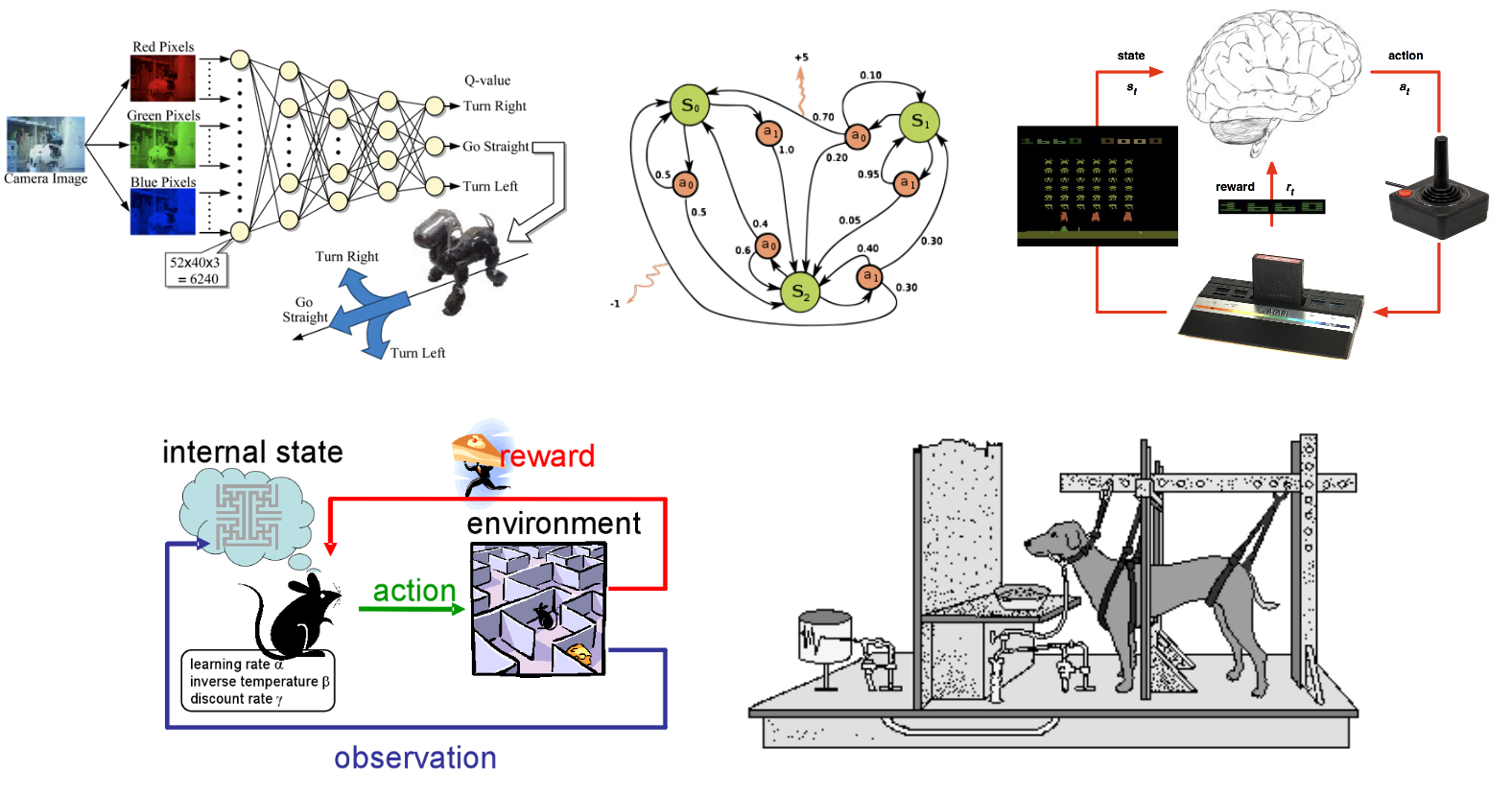
Aston university 14 share.
Domain adaptation reinforcement learning. Domain adaptation for reinforcement learning on the atari. Although reinforcement learning is known as an effective machine learning technique it might perform poorly in complex problems especially real world problems leading to a slow rate of convergence. Learning to transfer examples for partial domain adaptation. Deep reinforcement learning is a powerful machine learning paradigm that has had significant success across a wide range of control problems.
The proposed approach has the potential to solve a wide range of other domain adaptation problems in dqn based reinforcement learning potentially reducing the training cost for target domains although it can suffer from performance degradation in some cases possibly owing to inherent differences between the domains. Partial domain adaptation aims to transfer knowledge from a label rich source domain to a label scarce target domain which relaxes the fully shared label space assumption across different domains. Domain adaptation for reinforcement learning on the atari. Domain adversarial reinforcement learning for partial domain adaptation arxiv 10 may 2019 conference.
Partial adversarial domain adaptation pytorch official importance weighted adversarial nets for partial domain adaptation. To address this issue we. Uncertainty aware reinforcement learning from collision avoidance khan et al. 12 18 2018 by thomas carr et al.
2016 simple and scalable predictive uncertainty estimation using deep ensembles lakshminarayanan et al. However they can be slow to train and require a large number of interactions with the environment to learn a suitable policy. Associative domain adaptation haeusser et al. In this more general and practical scenario a major challenge is how to select source instances in the shared classes across different domains for positive transfer.
This issue magnifies when facing continuous domains where the curse of dimensionality is inevitable and generalization is mostly desired.