Domain Adaptation Remote Sensing
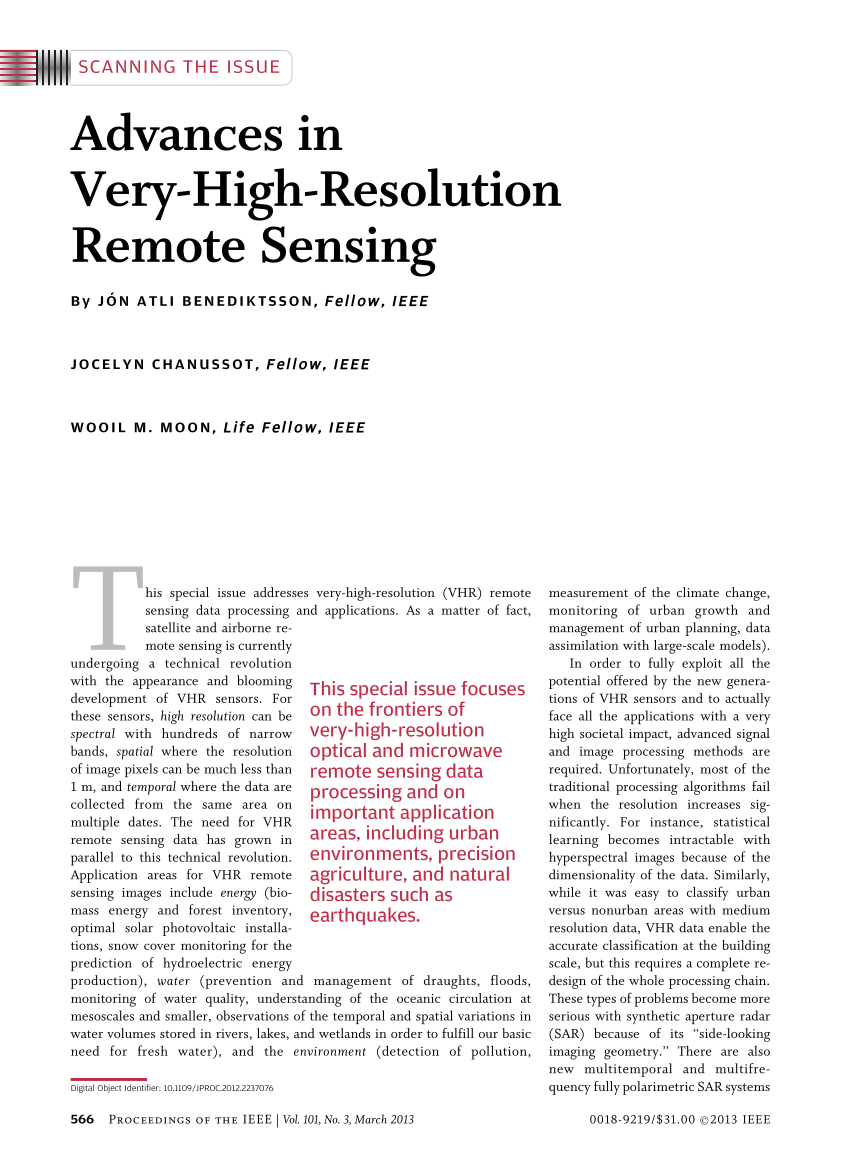
Lately domain adaptation is brought in remote sensing to solve these obstructive problems for classification.
Domain adaptation remote sensing. An overview of recent advances abstract. A novel efficient scalable yet simple adaptive multi source domain adaptation amda was developed to address this problem. In remote sensing data classification. Amda was also capable of dealing more effectively with the imbalanced data distribution among the sources.
Classification of remote sensing data. 1 invariant feature selection 2 representation matching 3 adaptation of classifiers and 4 selective sampling. A low rank reconstruction and instance weighting label propagation inspired algorithm qian shi bo du senior member ieee and liangpei zhang senior member ieee abstract this paper presents a framework for a semisuper vised domain adaptation method for remote sensing image clas. With the rapid development of the rs techniques a large number of rs scene images are available.
Download citation deep neural network based domain adaptation for classification of remote sensing images we investigate the effectiveness of deep neural network for cross domain. 8 august 2019 domain adaptation for convolutional neural networks based remote sensing scene classification shaoyue song hongkai yu zhenjiang miao member ieee qiang zhang yuewei lin and song wang senior member ieee abstract remote sensing rs scene classification. Multi temporal and multi source remote sensing image classification by nonlinear relative normalization. This article provides a critical review of the recent advances in da approaches for remote sensing and presents an overview of da methods divided into four categories.
The success of the supervised classification of remotely sensed images acquired over large geographical areas or at short time intervals strongly depends on the representativity of the samples used to train the classification algorithm and to define the model. Home knowledge base archives. Classification of hyperspectral images. 1 we try to implement this method in remote sensing classification to solve the existed problem that training and test data distribution have distinct difference for the model.
As manually labeling large scale rs scene images is both labor and time consuming when a new unlabeled data set is obtained how to use the existing labeled data sets to classify the new. Second the multi source domain adaptation for large scale applications was addressed. 1324 ieee geoscience and remote sensing letters vol. The comparative experiments demonstrate that the proposed da network outperforms the compared state of the art domain adaptation methods on remote sensing image scene classification.
Remote sensing rs scene classification plays an important role in the field of earth observation.